The 2020 European Conference on Computer Vision (ECCV’20) has accepted papers from several of our UW Reality Lab researchers: Reconstructing NBA Players; People As Scene Probe; and Lifespan Age Transformation Synthesis (all listed below). These papers will be part of the August 23-28 conference, being held entirely online due to the covid-19 pandemic. ECCV is “the top European conference in the image analysis area.”
Links to the project sites are below, along with abstracts, links, videos, and code – where available.
Congratulations to all these researchers!
Lifespan Age Transformation Synthesis
Roy Or-El, Soumyadip Sengupta, Ohad Fried, Eli Shechtman, Ira Kemelmacher-Shlizerman
CODE — COLAB DEMO — DATA
Abstract: We address the problem of single photo age progression and regression—the prediction of how a person might look in the future, or how they looked in the past. Most existing aging methods are limited to changing the texture, overlooking transformations in head shape that occur during the human aging and growth process. This limits the applicability of previous methods to aging of adults to slightly older adults, and application of those methods to photos of children does not produce quality results. We propose a novel multi-domain image-to-image generative adversarial network architecture, whose learned latent space models a continuous bi-directional aging process. The network is trained on the FFHQ dataset, which we labeled for ages, gender, and semantic segmentation. Fixed age classes are used as anchors to approximate continuous age transformation. Our framework can predict a full head portrait for ages 0–70 from a single photo, modifying both texture and shape of the head. We demonstrate results on a wide variety of photos and datasets, and show significant improvement over the state of the art.
People as Scene Probes
Yifan Wang, Steve Seitz, Brian Curless
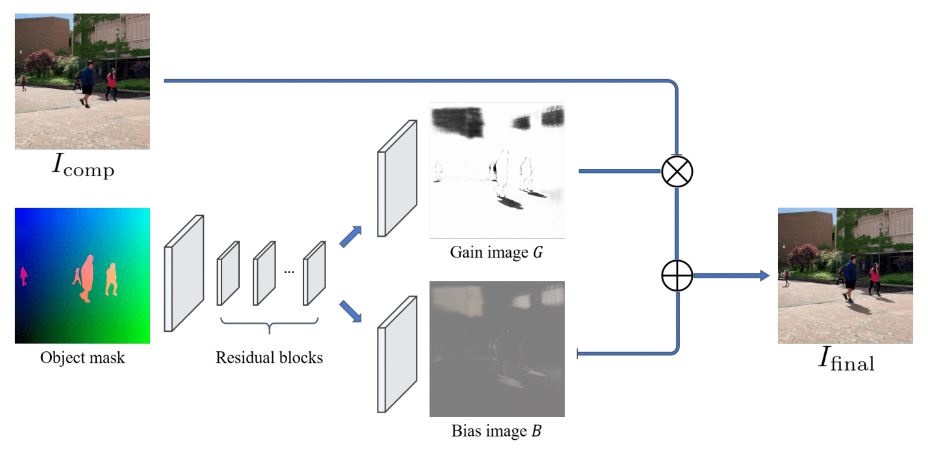
Abstract: By analyzing the motion of people and other objects in a scene, we demonstrate how to infer depth, occlusion, lighting, and shadow information from video taken from a single camera viewpoint. This information is then used to composite new objects into the same scene with a high degree of automation and realism. In particular, when a user places a new object (2D cut-out) in the image, it is automatically rescaled, relit, occluded properly, and casts realistic shadows in the correct direction relative to the sun, and which conform properly to scene geometry. We demonstrate results (best viewed in supplementary video) on a range of scenes and compare to alternative methods for depth estimation and shadow compositing.
Reconstructing NBA Players
Luyang Zhu, Konstantinos Rematas, Brian Curless, Steve Seitz, Ira Kemelmacher-Shlizerman
Abstract: Great progress has been made in 3D body pose and shape estimation from a single photo. Yet, state-of-the-art results still suffer from errors due to challenging body poses, modeling clothing, and self occlusions. The domain of basketball games is particularly challenging, as it exhibits all of these challenges. In this paper, we introduce a new approach for reconstruction of basketball players that outperforms the state-of-the-art. Key to our approach is a new method for creating poseable, skinned models of NBA players, and a large database of meshes (derived from the NBA2K19 video game), that we are releasing to the research community. Based on these models, we introduce a new method that takes as input a single photo of a clothed player in any basketball pose and outputs a high resolution mesh and 3D pose for that player. We demonstrate substantial improvement over state-of-the-art, single-image methods for body shape reconstruction.